The Comprehensive Guide to Annotated Image Datasets
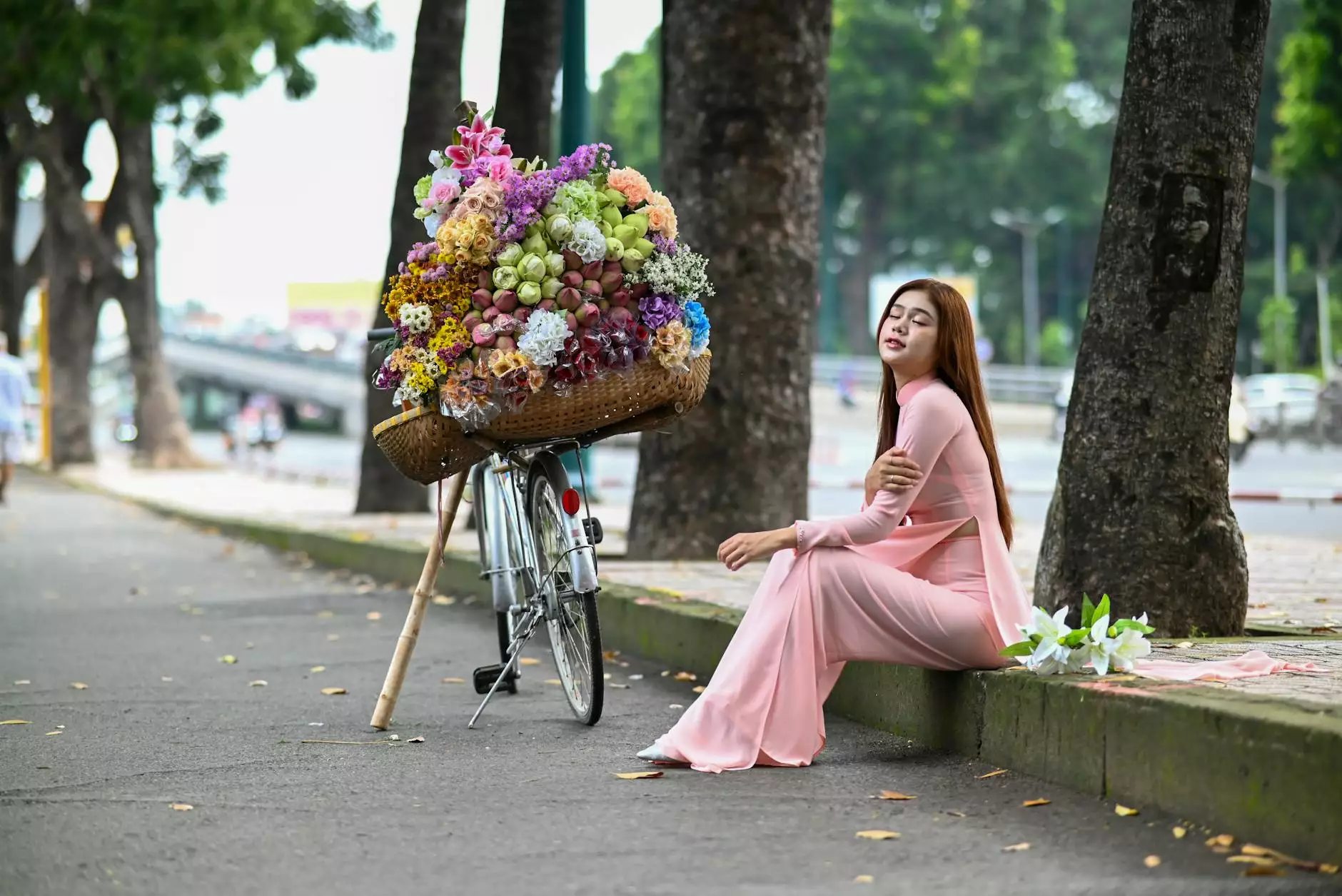
Introduction to Annotated Image Datasets
In the rapidly evolving realm of artificial intelligence (AI) and machine learning (ML), the role of annotated image datasets has become increasingly pivotal. These datasets serve as the backbone of training algorithms, enabling machines to learn from labeled examples and deliver accurate predictions. But what exactly are annotated image datasets, and why are they integral to the success of AI projects? This article delves deep into the world of data annotation, highlighting its value, the tools available, and how KeyLabs.ai stands at the forefront of this industry.
What is an Annotated Image Dataset?
An annotated image dataset is a collection of images that have been labeled with tags or descriptions to provide contextual information. This annotation enables algorithms to understand the content of the images and make informed decisions based on the learned data. The annotated data is often used to train machine learning models in various applications, from facial recognition systems to autonomous vehicles.
Types of Annotations
The annotations can vary widely depending on the application and desired outcome. Common types of annotations include:
- Bounding Boxes: Rectangular boxes drawn around objects to identify and localize them within an image.
- Segmentation: Pixel-level labeling that allows for detailed object recognition within images.
- Landmarks: Specific points labeled on objects, often used for facial recognition or anatomical studies.
- Textual Descriptions: Written descriptions that summarize the content or context of an image.
The Importance of Annotated Image Datasets in AI
Machine learning models, particularly those in computer vision, rely heavily on annotated image datasets to function correctly. The accuracy of a model directly correlates with the quality and quantity of the training data. Here are some key reasons why these datasets are so crucial:
1. Enhancing Model Accuracy
Well-annotated datasets enable machine learning models to grasp intricate patterns and correlations within the data. The more diverse and comprehensive the dataset, the better the model can generalize and make accurate predictions in real-world scenarios.
2. Reducing Bias in AI
Diversity in annotated datasets ensures that AI systems are less likely to exhibit bias. By incorporating a wide range of images from different demographics, backgrounds, and contexts, developers can create more equitable and fair AI solutions.
3. Improving Training Efficiency
High-quality annotations can significantly reduce the training time for models. When data is clearly labeled, algorithms can learn faster, leading to quicker iterations and more efficient development cycles.
The Role of Data Annotation Tools and Platforms
To harness the power of annotated image datasets, businesses often rely on data annotation tools and platforms. These tools streamline the process of labeling images, making it easier to produce high-quality annotated datasets. Here are several features and benefits of modern data annotation tools:
1. User-Friendly Interfaces
Leading data annotation platforms come equipped with intuitive interfaces that simplify the annotation process. Drag-and-drop functionality, shortcut keys, and clear prompts enhance user experience, making it accessible even for those with minimal technical expertise.
2. Automation Features
Many state-of-the-art annotation tools offer automation capabilities such as pre-labeling, where an AI algorithm suggests labels based on existing data. This can drastically reduce the time required for manual annotation while maintaining accuracy.
3. Collaboration and Scalability
Cloud-based annotation platforms allow teams to collaborate in real-time and scale their efforts as project demands grow. This means that businesses can handle larger datasets without sacrificing the quality of annotations.
KeyLabs.ai: Transforming Data Annotation
KeyLabs.ai leverages cutting-edge technology to streamline the process of data annotation, particularly for annotated image datasets. With a focus on quality assurance, our platform enables users to produce highly accurate and reliable datasets crucial for successful machine learning applications.
Features of KeyLabs.ai
Here are several features that make KeyLabs.ai a leader in the data annotation space:
- Comprehensive Toolsets: A variety of annotation tools including bounding box, segmentation, and landmark detection.
- AI-Assisted Annotation: Utilize AI to expedite the annotation process, allowing for faster turnarounds without compromising quality.
- Robust Quality Control: Rigorous review processes ensure high accuracy rates in annotations.
- Custom Solutions: Tailored annotation services to fit the unique needs of different projects and industries.
Understanding the Impact of Annotated Image Datasets
As industries increasingly adopt AI technologies, the impact of correctly annotated image datasets cannot be overstated. Here, we explore how various sectors benefit from these datasets.
1. Healthcare
In healthcare, annotated image datasets play a critical role in train machine learning algorithms for diagnostic purposes. Medical imaging, such as MRI and CT scans, relies on accurate annotations to help in identifying patterns related to conditions such as tumors.
2. Autonomous Vehicles
The autonomous vehicle industry requires extensive annotated image datasets to train models for recognizing various objects on the road, including pedestrians, traffic signs, and other vehicles. High-quality annotations ensure safety and reliability in navigation systems.
3. Retail and E-commerce
E-commerce companies utilize annotated datasets to improve visual search capabilities. By training models on labeled product images, businesses can enhance their recommendation systems and improve user experience.
How to Build Your Own Annotated Image Dataset
For businesses looking to develop proprietary models, building a custom annotated image dataset may be necessary. The following steps outline how to achieve this:
1. Define Your Goals
Clearly outline the objectives of your dataset. Determine what you need the dataset for and how it aligns with your overall project goals.
2. Collect Data
Gather a diverse set of images relevant to your use case. The quantity and diversity of images play a crucial role in the dataset's effectiveness.
3. Annotation
Utilize a robust annotation tool, like KeyLabs.ai, to ensure accuracy and efficiency in labeling your images. Consider involving domain experts for more complex annotations.
4. Quality Assurance
Implement a quality assurance process to review annotations for accuracy. This could involve multiple rounds of review or automated checks for consistency.
5. Testing and Validation
Test your model using the annotated dataset and validate its performance. Make any necessary adjustments to improve accuracy.
Conclusion
In conclusion, annotated image datasets are essential for the success of machine learning models across various domains. The quality of these datasets plays a significant role in determining the effectiveness of AI systems. By utilizing advanced data annotation tools and platforms like KeyLabs.ai, businesses can elevate their projects and achieve greater accuracy and performance. As AI continues to evolve, the demand for high-quality annotated datasets will only grow, making it imperative for organizations to invest in this vital component of AI development.