Unlocking the Potential of Image Annotation in Machine Learning
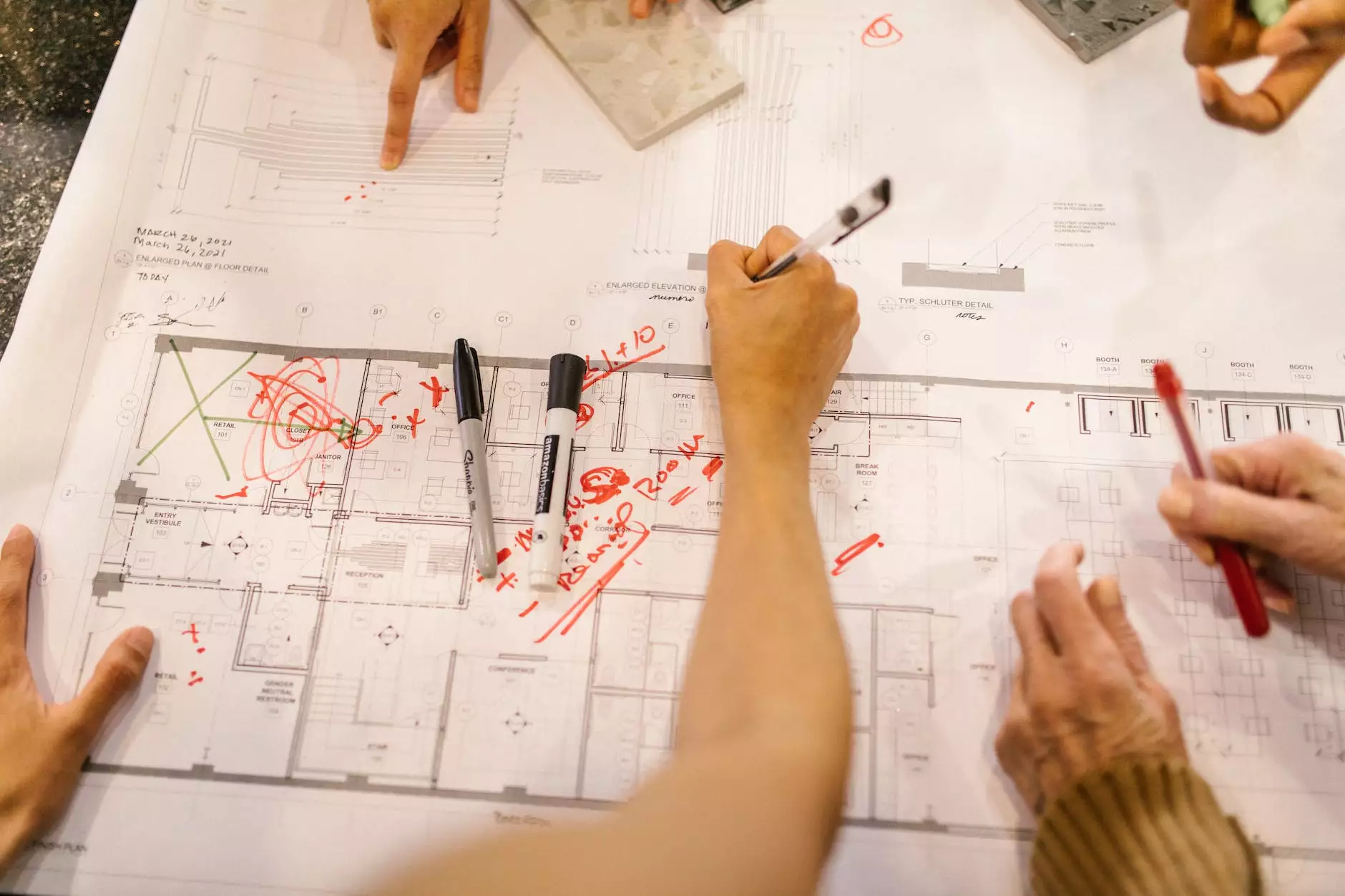
The world of machine learning is rapidly evolving, with businesses across various sectors leveraging its power to improve their services, enhance customer experience, and drive innovation. One of the most critical elements in developing machine learning models is image annotation, a process that enables machines to understand visual data through labeling.
Understanding Image Annotation
Image annotation is the technique of labeling images to provide context and meaning to the data contained within them. In the realm of machine learning, this process is essential for training algorithms to recognize patterns and make predictions based on visual inputs. Typically, image annotation involves assigning tags, shapes, or bounding boxes to images, allowing algorithms to learn from the labeled data. This is fundamental in various applications, from self-driving cars to facial recognition software.
How Image Annotation Fuels Machine Learning
Without image annotation, machine learning models would lack the necessary data to improve their functionality. The following outlines the major contributions of image annotation to machine learning:
- Data Quality Improvement: Quality annotated data boosts the performance and accuracy of machine learning models.
- Training Supervised Learning Models: Most machine learning applications, especially in vision, depend on supervised learning where annotated data is critical.
- Facilitating Real-World Applications: From healthcare diagnostics using image recognition to enhancing security systems through facial recognition, annotated images empower various industries.
Applications of Image Annotation in Business
Various industries benefit from image annotation, including:
1. Automotive Industry
In the automotive sector, image annotation is vital for the development of autonomous vehicles. Annotated datasets help self-driving cars learn to identify pedestrians, other vehicles, traffic signals, and more. The precision of these annotations ensures the safety and efficiency of vehicle navigation systems.
2. Retail and E-commerce
In retail, businesses use image annotation to improve their product search functionalities. By annotating images, machine learning algorithms can accurately classify products, making it easier for customers to find what they’re looking for, enhancing their shopping experience.
3. Healthcare Sector
Healthcare applications utilize image annotation in analyzing medical images such as MRIs and X-rays. Annotated datasets enable machine learning models to detect anomalies, predict diseases, and provide insights that aid medical professionals in making better-informed decisions.
4. Security and Surveillance
In security systems, image annotation helps in identifying and classifying individuals and objects in surveillance footage. This enhances the ability of surveillance systems to promptly alert personnel to suspicious activities, thereby improving safety measures.
The Role of Annotation Services in Image Annotation
Many companies opt for professional image annotation services to streamline this critical process. These services employ skilled annotators and leverage advanced tools to ensure high-quality annotation. By outsourcing this task, businesses can focus on their core activities while ensuring that their datasets are well-prepared for machine learning.
Benefits of Using Professional Annotation Services
- Scalability: Services can handle large volumes of images quickly, making them suitable for businesses of all sizes.
- Consistency: Professional annotators follow strict guidelines to ensure that data labeling is uniform and standardized.
- Quality Assurance: Companies provide quality checks and balances, ensuring high accuracy in annotated data.
Best Practices in Image Annotation
For businesses looking to perform their own image annotation, several best practices can help improve the process:
- Define Clear Objectives: Understand the purpose of the annotation. Different projects may require different labeling strategies.
- Use the Right Tools: Invest in specialized software that facilitates easy and efficient annotation.
- Regular Training: Ensure annotators are well-trained in the guidelines and tools used for annotation to maintain data quality.
- Feedback Loop: Implement a system for continuous feedback to improve the quality of annotations over time.
The Future of Image Annotation and Machine Learning
As technologies continue to advance, the future of image annotation in machine learning looks promising. Emerging trends suggest that:
1. Increased Automation
Tools that leverage artificial intelligence for pre-annotation are emerging, streamlining the process and reducing human effort.
2. Enhanced Quality Control
With the rise of sophisticated algorithms, machine learning may improve the quality-checking processes for annotated datasets.
3. Expansion of Use Cases
The applications of image annotation will continue to grow, penetrating more sectors and leading to innovative business solutions.
Conclusion
In conclusion, image annotation serves as a backbone for machine learning development across various industries. As businesses like KeyMakr continue to explore the integration of machine learning into their operations, mastering the art of image annotation can unlock new potentials for enhancing services such as locksmithing. With significant advancements in technology, understanding and applying effective image annotation strategies will remain essential for businesses aiming to stay competitive in a rapidly evolving digital landscape.
As we move forward, investing in quality image annotation practices will not only drive the success of machine learning initiatives but also revolutionize the way we perceive and interact with technology in daily life.
image annotation machine learning