Unlocking Business Success Through Data Labeling
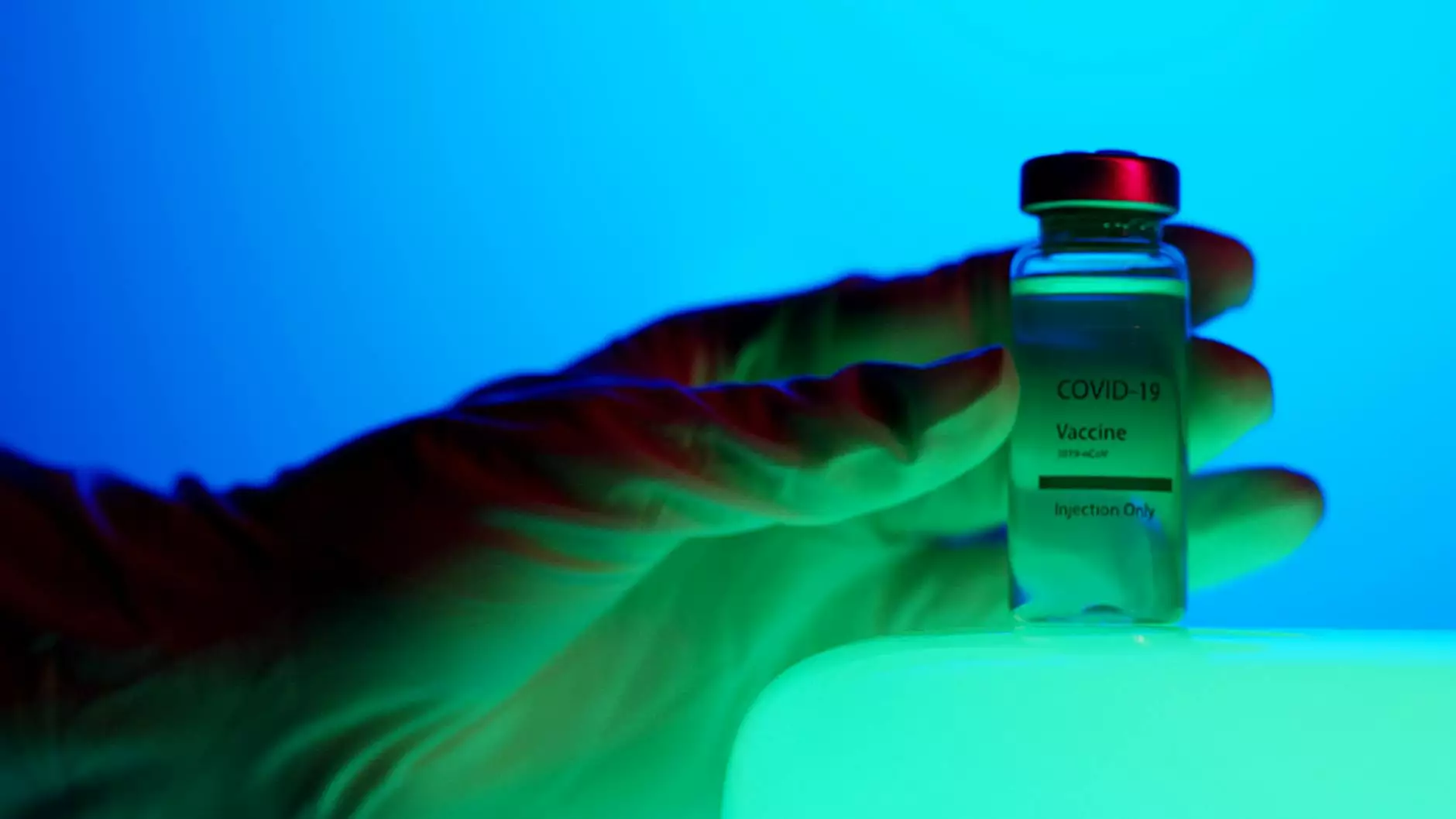
Data labeling is a critical component in today’s digital landscape, especially as businesses are increasingly relying on artificial intelligence (AI) and machine learning (ML) technologies. As organizations strive to harness the potential of data, understanding the significance of managing and annotating that data becomes imperative. This article delves into the multifaceted world of data labeling, highlighting its benefits, methodologies, and how it can elevate your business strategies through enhanced data annotation tools and platforms provided by Key Labs AI.
What is Data Labeling?
Data labeling is the process of annotating data to create a structured dataset that can be used for training machine learning models. This can involve tagging images, transcribing audio files, or labeling text for sentiment analysis, among other tasks. Data labeling enables machines to understand and interpret vast amounts of information, thereby allowing the AI to learn patterns and make informed predictions.
The Importance of Data Labeling for Businesses
In an era where data drives decision-making, the importance of data labeling cannot be overstated. Here are several key reasons why businesses should prioritize this process:
- Improved Accuracy: Properly labeled data ensures that machine learning algorithms can produce accurate outputs, which is vital for applications ranging from image recognition to natural language processing.
- Enhanced Decision Making: Accurate data labeling can lead to better insights and analytics, empowering businesses to make informed decisions based on reliable data.
- Competitive Edge: Organizations that effectively implement data labeling gain a competitive advantage by utilizing cutting-edge technologies that rely on well-structured data.
- Increased Efficiency: Automated data labeling systems streamline the annotation process, saving time and resources while enabling teams to focus on strategic tasks.
Understanding Different Types of Data Labeling Techniques
There are several techniques employed in data labeling that businesses can leverage, each catering to specific types of data and operational requirements:
1. Image Annotation
Image annotation involves the process of labeling images to provide training datasets for computer vision models. Common techniques include:
- Bounding Boxes: A rectangular box is drawn around objects of interest within the image.
- Polygon Segmentation: Polygons are used to outline the exact shape of an object for more precision.
- Semantic Segmentation: Each pixel in the image is classified into predefined categories.
2. Video Annotation
Video annotation is similar to image annotation but involves labeling video frames. This process is vital for applications in surveillance, autonomous vehicles, and sports analytics. Techniques include:
- Frame-by-Frame Labeling: Each frame is annotated with relevant data.
- Object Tracking: Continuous tracking of objects across multiple frames.
3. Text Annotation
Text annotation involves labeling textual data for various purposes such as sentiment analysis, entity recognition, and more. Key methods include:
- Sentiment Labeling: Determining the emotional tone behind a series of words.
- Entity Recognition: Identifying specific entities within the text, such as names, dates, and locations.
How to Implement an Effective Data Labeling Strategy
Implementing a successful data labeling strategy requires careful planning and consideration. The following steps can guide businesses in establishing an effective approach:
1. Define Your Goals
Before starting the data labeling process, clearly define the objectives you aim to achieve with your machine learning models. This might involve improving customer service with chatbots or increasing accuracy in image recognition tasks.
2. Choose the Right Tools and Platforms
Selecting a robust data annotation tool or platform is crucial for efficiency and accuracy. Platforms like Key Labs AI offer comprehensive solutions for various annotation needs.
3. Establish Data Quality Standards
Set clear quality standards to ensure that the labeled data meets the required specifications for your AI models. This may include proper guidelines for annotators and a review process to verify the accuracy of the annotations.
4. Leverage Automation
Incorporate automated data labeling processes where possible to enhance speed and reduce manual errors. Automation can significantly cut down the time needed for data preparation.
5. Continuous Monitoring and Assessment
Continuously monitor the performance of your data labeling efforts and assess the results generated by your machine learning models. Incorporating feedback loops will help improve future labeling tasks.
Benefits of Using Advanced Data Annotation Platforms
Utilizing robust data annotation platforms, like the ones provided by Key Labs AI, comes with a multitude of advantages:
- Scalability: Handle large volumes of data efficiently without compromising on quality.
- Customization: Platforms allow for tailored annotation processes that suit specific business needs.
- Collaboration: Facilitate teamwork among data scientists, annotators, and project managers, ensuring everyone is aligned on the goals.
- Real-Time Updates: Keep data up to date and relevant, enabling quick iterations based on new data.
Challenges in Data Labeling and How to Overcome Them
Despite the numerous benefits, businesses may encounter challenges in the data labeling process. Identifying these challenges and knowing how to address them is essential for success:
1. High Labor Cost
The manual labeling process can be resource-intensive. To mitigate costs, consider integrating automated tools that can perform initial labeling with human review to ensure accuracy.
2. Quality Control Issues
Maintaining high-quality standards is paramount in data labeling. Employ systematic reviews and continuous training for annotators to improve accuracy rates.
3. Rapidly Changing Data
Data trends evolve, requiring regular updates to the labeled datasets. Establishing a routine for re-evaluating and updating annotations can help keep your data relevant.
Conclusion: The Future of Data Labeling in Business
As businesses continue to recognize the power of data, embracing effective data labeling practices will become increasingly vital. The potential applications of properly labeled data are vast, ranging from customer service improvements to advancements in healthcare and beyond. With companies like Key Labs AI at the forefront, providing sophisticated data annotation tools and platforms, organizations can unlock new avenues for growth and innovation.
In conclusion, investing in quality data labeling processes is not just a necessity; it is a strategic move that enables companies to harness the full potential of their data, ultimately driving business success. By defining clear objectives, choosing the right tools, and implementing efficient strategies, businesses can stay ahead in an ever-evolving digital landscape.